In today’s fast-paced and ever-changing global economy, staying ahead of market trends is crucial for businesses and investors. Traditional methods of market analysis, while valuable, often struggle to keep pace with the sheer volume of data generated daily. This is where Artificial Intelligence (AI) steps in, offering powerful tools to predict market trends with unprecedented accuracy and speed.
This blog explores how AI is revolutionizing market forecasting, its applications across industries, the technologies driving it, and the challenges involved.
What is Market Trend Prediction?
Market trend prediction involves analyzing data to identify patterns and forecast future movements in markets. These trends may include changes in consumer behavior, stock prices, economic indicators, or industry-specific shifts.
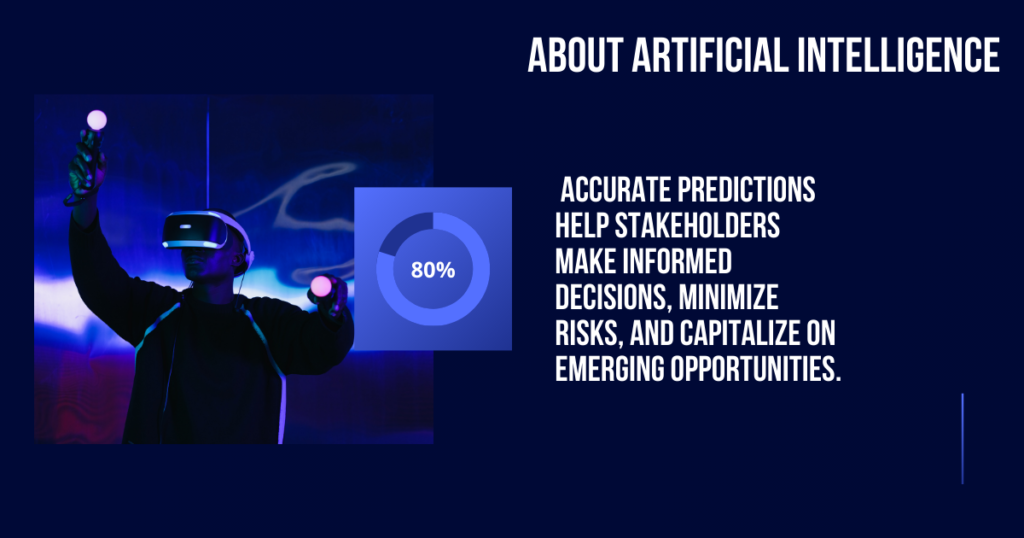
Traditional market analysis methods rely on statistical models, historical data, and expert judgment. However, they are limited by human biases and the inability to process massive datasets. AI overcomes these limitations by leveraging advanced algorithms and computing power to extract actionable insights from complex data.
How AI Predicts Market Trends
AI employs various technologies to analyze data, detect patterns, and generate forecasts. The key components include:
1. Machine Learning (ML)
Machine learning algorithms learn from historical data to identify patterns and make predictions. In market trend prediction, ML models analyze factors such as:
- Stock price movements
- Consumer sentiment
- Macroeconomic indicators
For instance, ML models can use regression techniques to forecast sales or classify stocks as bullish or bearish based on past performance.
2. Natural Language Processing (NLP)
NLP allows AI systems to analyze and interpret human language. It’s particularly useful for processing unstructured data like news articles, social media posts, and analyst reports.
- Sentiment Analysis: Determines public sentiment about a company or product, influencing stock prices or consumer trends.
- Trend Detection: Identifies frequently discussed topics or keywords that signal emerging market interests.
3. Deep Learning (DL)
Deep learning uses neural networks to process large datasets with high complexity. Convolutional Neural Networks (CNNs) and Recurrent Neural Networks (RNNs) are commonly used for:
- Analyzing time-series data like stock prices or economic indicators.
- Predicting future outcomes based on historical trends.
4. Big Data Analytics
AI thrives on vast amounts of data. Big data platforms enable AI to analyze information from diverse sources, including:
- Transactional data
- Social media activity
- Satellite imagery (e.g., analyzing retail traffic or agricultural outputs)
5. Predictive Analytics
AI models combine historical data, current trends, and real-time inputs to create actionable predictions. Predictive analytics tools are widely used in finance, retail, and supply chain management.
Applications of AI in Market Trend Prediction
1. Financial Markets
AI has revolutionized trading and investment strategies by providing insights into market movements.
- Algorithmic Trading: AI-driven algorithms execute trades based on market conditions, improving efficiency and reducing emotional decision-making.
- Portfolio Management: Predictive models assess risks and recommend diversified portfolios tailored to individual goals.
- Stock Price Forecasting: AI analyzes historical price data, economic indicators, and sentiment to predict stock performance.
2. Retail and Consumer Trends
Understanding consumer behavior is critical for businesses aiming to stay competitive. AI helps retailers:
- Identify emerging product trends through social media analysis.
- Forecast demand for inventory management and supply chain optimization.
- Personalize marketing strategies based on customer preferences.
3. Real Estate
AI tools predict property values, rental trends, and market demand by analyzing data like economic growth, urban development, and interest rates. This aids real estate investors and developers in making data-driven decisions.
4. Supply Chain and Logistics
AI improves supply chain efficiency by forecasting demand fluctuations and identifying potential disruptions. For example:
- Predicting demand spikes during holiday seasons.
- Optimizing delivery routes based on real-time data.
5. Energy and Commodities
Energy markets are volatile, influenced by geopolitical events, weather patterns, and economic conditions. AI predicts:
- Crude oil prices based on historical trends and geopolitical news.
- Renewable energy production by analyzing weather forecasts and grid data.
Technologies Powering AI Market Predictions
1. Neural Networks
Neural networks process non-linear relationships in data, making them ideal for predicting complex market behaviors. Recurrent Neural Networks (RNNs) and Long Short-Term Memory (LSTM) networks are particularly effective for time-series forecasting.
2. Data Visualization Tools
AI integrates with visualization platforms to present predictions in user-friendly dashboards. These tools make it easier for decision-makers to interpret results and act on insights.
3. Cloud Computing
AI models often require significant computational power. Cloud platforms provide scalable resources for training and deploying AI systems without heavy upfront investment.
4. Blockchain for Data Integrity
Blockchain ensures the integrity of data used in AI models by providing a secure, tamper-proof environment. This is especially valuable in financial markets, where data accuracy is critical.
Case Studies: AI in Action
Case Study 1: Hedge Funds and AI
Several hedge funds now use AI to predict market trends. For example, Renaissance Technologies employs machine learning algorithms to analyze historical data and execute trades. Their AI-driven strategies have consistently outperformed traditional methods.
Case Study 2: Retail Giants
Amazon uses AI to forecast product demand, optimize inventory, and personalize recommendations. By analyzing millions of transactions daily, Amazon stays ahead of consumer trends and minimizes operational costs.
Case Study 3: Agriculture and Commodity Markets
AI-powered platforms like Descartes Labs analyze satellite imagery to predict crop yields and their impact on commodity prices. This information helps traders make informed decisions.
Challenges in Using AI for Market Predictions
1. Data Quality and Availability
AI models rely on high-quality data for accurate predictions. Incomplete, biased, or outdated data can lead to errors. Ensuring diverse and representative datasets is crucial.
2. Interpretability
Many AI models, especially deep learning networks, operate as “black boxes,” making their decision-making process opaque. This lack of transparency can hinder trust and adoption.
3. Overfitting
AI models can sometimes become too specialized in their training data, making them less effective in predicting new or unseen scenarios.
4. Ethical Concerns
AI systems can perpetuate biases present in training data, leading to unfair outcomes. For instance, biased sentiment analysis might favor certain demographic groups over others.
5. Regulatory Compliance
The use of AI in finance and other regulated industries must adhere to strict compliance standards. Violations can result in significant penalties.
The Future of AI in Market Trend Prediction
The future of AI in market prediction is bright, with several trends shaping its evolution:
- Integration with Quantum Computing: Quantum computing promises to accelerate AI’s data processing capabilities, enabling more accurate and faster predictions.
- Explainable AI (XAI): Efforts to make AI systems more transparent and interpretable will increase trust and adoption.
- AI-Powered Marketplaces: Platforms like OpenAI’s Codex could democratize access to market prediction tools, enabling smaller businesses to compete.
- Real-Time Predictions: Advancements in real-time data processing will make predictions even more dynamic and actionable.
Conclusion
AI is transforming how businesses and investors predict market trends, providing insights that were previously unimaginable. From financial markets to retail, real estate, and energy, AI-driven predictions empower stakeholders to make better decisions, mitigate risks, and seize opportunities.
While challenges like data quality, interpretability, and ethical concerns persist, continuous advancements in AI technologies are addressing these issues. As AI becomes more sophisticated, its role in market trend prediction will only grow, making it an indispensable tool in the modern economy.
By leveraging AI responsibly, businesses and individuals can not only stay ahead of the curve but also contribute to a more informed and equitable market landscape.